Changing atmospheric blocking; new insights with novel machine learning detection
Elina
Valkonen
ESSIC UMD / NASA GSFC
Poster
Atmospheric blocking plays a role in defining the general circulation pattern and contributes to many extreme weather events, such as atmospheric rives or heat waves. Accurately detecting atmospheric blocks from climate model data is important, as it will help the study and prediction of blocking in changing climate. We will present results of current and future blocking climatologies across all CMIP6 data available at PCMDI for the historical and future scenario simulations (1979-2010, 2014-2100). These climatologies will provide more robust understanding of our abilities in modeling atmospheric blocks and give us more in-depth understanding of future changes given the vast amount of data used to produce these climatologies. Results will be split seasonally, and emphasis will be given to future changes and their robustness across the model ensembles and different scenarios.
We will also discuss the development of the blocking algorithm, which is a global ML-based blocking detection algorithm we created. The ML model was trained on binary labels generated with the TempestExtremes tracking algorithm for three separate CMIP6 models. The model was trained with daily 500 hPa geopotential height fields from 1950-2100 to guarantee the generalization capabilities of the model in the changing climate. The ML detection algorithm’s performance will be shown for individual block detection, seasonal climatologies, and standard ML performance metrics. Discussion will also briefly touch on the benefits and possible future uses for ML in detection of extreme events.
We will also discuss the development of the blocking algorithm, which is a global ML-based blocking detection algorithm we created. The ML model was trained on binary labels generated with the TempestExtremes tracking algorithm for three separate CMIP6 models. The model was trained with daily 500 hPa geopotential height fields from 1950-2100 to guarantee the generalization capabilities of the model in the changing climate. The ML detection algorithm’s performance will be shown for individual block detection, seasonal climatologies, and standard ML performance metrics. Discussion will also briefly touch on the benefits and possible future uses for ML in detection of extreme events.
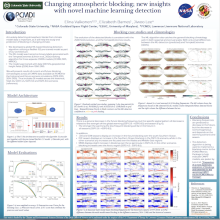
Poster file
Valkonen_Elina_Blocking_poster.pdf
(5.96 MB)
Meeting homepage