Preparation of a more systematic evaluation of blockings and extremes for the upcoming CMIP7 via the PCMDI Metrics Package
Jiwoo
Lee
Lawrence Livermore National Laboratory
Poster
Systematic and collective evaluations and benchmarking of climate models have become more and more important with the exponential growth of climate simulations. The most recent phase of the Coupled Model Intercomparison Project, CMIP6, provides a set of well-defined experiments that most climate modeling centers perform, subsequently making results available for a large and diverse community to analyze. Model output of the CMIP6 is at the Petabyte scale and it is expected that the next phase (CMIP7) will be even more extensive. With that, there has been a pressing need for the research community to become more efficient and systematic in evaluating ESMs and documenting their performances. To respond to the need, the Program for Climate Model Diagnosis and Intercomparison (PCMDI) has developed the PCMDI Metrics Package (PMP). The PMP is an open-source Python software package that meets the challenge of model evaluation by providing "quick-look" objective comparisons of ESMs with one another and with observations, and it is expected to play an important role in benchmarking the upcoming CMIP7. To consider blocking and extremes in the systematic evaluation workflow in addition, we have implemented extreme metrics and shaped plans to implement atmospheric blocking metrics. The blocking metrics were developed using a deep learning algorithm trained on CMIP6 models and TempestExtremes. For extreme metrics, extreme daily precipitation and temperature indices and their long-period (e.g., 20-year) return values are calculated using a non-stationary Generalized Extreme Value statistical method. In this presentation, we will introduce the PMP and its progress in applying the extreme and blocking metrics.
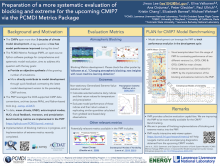
Poster file
Lee_Jiwoo_blocking_poster.pdf
(4.16 MB)
Meeting homepage