Detection of anthropogenic influence on the historical evolution of annual extreme rainfall
Celine
Bonfils
Laurence Livermore National Laboratory
Poster
The evolution of Earth's climate is shaped by the superimposition of natural forcings, such as variations in solar radiation and volcanic activity, and human forcings, including the increase in greenhouse gas concentrations and the emission of particulate pollution, onto sequences of internal climate variability. Aside from model errors and uncertainties in observations and forcings, the divergence between simulated and observed trends often arises from the different manifestation of climate variability, i.e. the fluctuations in climate that are not directly attributed to external forcings. Assessing the climate models’ ability to reproduce the forced component of observed trends can be greatly facilitated by the application of traditional and machine learning fingerprinting methods, and by the utilization of large ensembles (composed of ensemble members sharing the same forced signal on top of random and uncorrelated sequences of internal variability). In this presentation, we will illustrate how fingerprinting-based analyses can aid in achieving the estimated partitioning of forced and unforced components in observations. We will also show how fingerprinting methods can be used to assess the consistency between forced simulated trends and observational data.
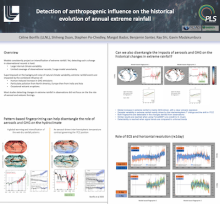
Poster file
bonfils-celine-confronting-poster.pdf
(9.65 MB)