Evaluating Earth System Models Against Observations using Machine Learning Assisted Analog Forecasting
Martin
Fernandez
Colorado State University
Poster
Multi-year to decadal climate predictions are key tools in understanding the range of potential futures of regional and global climate. Here, a framework that uses a neural network derived weighted mask to select model analogs for multi-year to decadal climate predictions is presented. Analog forecasting is the method of finding the most similar climate states to the state of interest (SOI), and assuming these analog states will evolve similarly to the SOI, i.e. the prediction for the SOI is the known future of the analog states. The neural network contributes to this method by highlighting the regions that are most important in determining if two climate states will evolve similarly, ensuring that the analog selection encodes information specific to the prediction task.
The library of potential analogs used includes 30 CMIP6 Earth system models and the SOI is from observations. The mean and spread of the top analogs, selected by their mean squared error relative to the SOI, act as the prediction and prediction uncertainty. By providing a large library that includes many different ESMs, the analog selection process acts as a measure of how well each ESM in the library recreates the SOI for the given variable, time scale, and region being predicted.
I will present results highlighting how much these ESMs lead or lag the temperature and precipitation trend as compared to observations (BEST, ERA5), how the ESM analog selection evolves with time (which models do best, and when), and how often these ESMs are selected as analogs (a proxy for how well they model the observations). For the regions and variables explored, some ESMs are selected much more often than others, even when accounting for the different number of ensemble members available for each ESM. Additionally, the learned mask allows us to investigate what regions were important in determining whether an ESM provided a “good” or “bad” representation of the observations. The framework can be applied to any global region, and is robust on time scales from 1 to 10 years, making it a useful tool for quantifying where specific ESMs could be improved moving forward.
The library of potential analogs used includes 30 CMIP6 Earth system models and the SOI is from observations. The mean and spread of the top analogs, selected by their mean squared error relative to the SOI, act as the prediction and prediction uncertainty. By providing a large library that includes many different ESMs, the analog selection process acts as a measure of how well each ESM in the library recreates the SOI for the given variable, time scale, and region being predicted.
I will present results highlighting how much these ESMs lead or lag the temperature and precipitation trend as compared to observations (BEST, ERA5), how the ESM analog selection evolves with time (which models do best, and when), and how often these ESMs are selected as analogs (a proxy for how well they model the observations). For the regions and variables explored, some ESMs are selected much more often than others, even when accounting for the different number of ensemble members available for each ESM. Additionally, the learned mask allows us to investigate what regions were important in determining whether an ESM provided a “good” or “bad” representation of the observations. The framework can be applied to any global region, and is robust on time scales from 1 to 10 years, making it a useful tool for quantifying where specific ESMs could be improved moving forward.
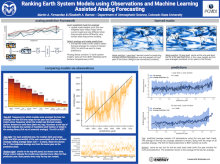
Poster file
fernandez-martin-confronting-poster.pdf
(2.66 MB)