Predicting the Trend in Real Time: Climate Change Aware Bias Corrections of Dynamical Models in S2S Forecasting
Dan
Collins
NOAA/NWS/NCEP Climate Prediction Center
Poster
Dynamical models and multi-model ensembles (e.g., North American Multi-Model Ensemble, Copernicus) are a primary tool for the prediction of temperature and precipitation on seasonal timescales. Bias correction and calibration of model derived probabilities are an essential component of the forecast process and known to improve skill. While post-processing typically derives statistics from multi-decadal hindcasts to apply to calibration of real-time forecasts, skill and biases may be conditionally dependent on the strength of climate signals from seasonal to decadal timescales. Decadal timescale climate changes are one of the primary sources of predictability on seasonal timescales. Moreover, there may be changes in the impacts of interannual climate phenomena, such as the El Nino Southern Oscillation (ENSO) over decadal timescales. In general, signals related to various climate forcings and timescales are all evaluated simultaneously in the validation and post-processing of seasonal forecasts. In this analysis, we analyze the skill and errors of dynamical models related to longer decadal timescales and shorter interannual timescales (excluding decadal variability). It is found that, depending on the season and region, much of the skill of model seasonal forecasts can be attributed to decadal timescale temperature trends. However, it is found also that in some seasons and locations, decadal variability is poorly represented by the seasonal model forecasts (including the shortest lead times of one to three months). Statistical correction of the decadal signals in seasonal forecasts is shown to improve skill on seasonal timescales. In addition, the explicit consideration of climate change in “trend-aware” calibration of dynamical model forecasts reveals that ensemble models have probabilistic skill that is independent of decadal timescale variability. However, much of the skill of S2S forecasts is attributable to trends. Determining climate trends from observations in real-time and biases in dynamical models related to trends is key to the continued advancement of seasonal forecasting during a period of accelerating climate change.
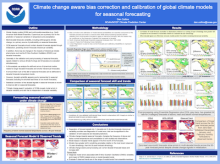
Poster file
collins-dan-confronting-poster.pdf
(1.64 MB)