Exploring parametric and structural uncertainties of bulk and superdroplet ice microphysical schemes in large eddy simulations
Joseph
Ko
Columbia University
Poster
Microphysical parameterizations contribute to large uncertainties in weather and climate models. Lagrangian superdroplet methods provide the most explicit and detailed microphysical representation of clouds in atmospheric models; however they are prohibitively expensive to run in the context of large-scale simulations (e.g., operational weather forecasting, global climate models). In this work, we use a collection of cloud-scale large eddy simulations (NCAR’s Cloud Model 1 i.e., CM1) using both bulk and superdroplet microphysics to probe underlying parametric and structural uncertainties of cirrus cloud process models. First, we use a large perturbed parameter ensemble (O(100)) of bulk large eddy simulations and analogous superdroplet-based simulations as higher-fidelity “ground truth” to estimate the optimal parameters and associated uncertainties for habit-dependent vapor depositional growth. Second, we diagnose underlying structural differences between bulk and superdroplet schemes that contribute to significant differences in model outputs, even within the same dynamical model and equivalent theoretical frameworks for microphysical processes. Finally, we will demonstrate how unsupervised representation learning can be leveraged to systematically compare model outputs in latent space, in the context of parameter estimation and model evaluation more broadly.
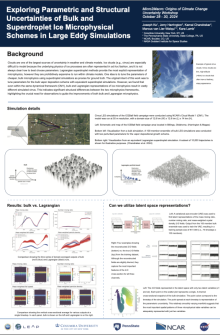
micro2macro_poster_2024_joseph_ko copy.pdf
(10.52 MB)
Meeting homepage