How well can power laws describe microphysical processes in bulk schemes?
Arthur Z.
Hu
Columbia University
Poster
Understanding and accurately simulating cloud microphysics is crucial for improving weather and climate models. Traditional bulk microphysical schemes, which parameterize microphysical processes using simplified relationships, often rely on power laws to calculate cloud condensation nuclei number and process rates such as collision-coalescence and sedimentation. However, the appropriateness of power laws for capturing the complex nature of these processes remains understudied. This work aims to evaluate the effectiveness of power-law based parameterizations in bulk microphysics schemes by comparing three different approaches: a bin scheme, the Arbitrary Moment Predictor (AMP), and the Bayesian Observationally Constrained Statistical–Physical Scheme (BOSS). The bin scheme provides detailed representation of the droplet size distribution (DSD) and its evolution, which serves as a benchmark for microphysics. BOSS uses Bayesian inference to learn the coefficients and powers in the power law process rate equations from the bin simulation results. AMP runs bin microphysics routines but only relays a set of moments (instead of the entire DSD) from the previous to the next time step, which represents a "bulkified" bin scheme and can serve as a reference for single time step process rate. In addition to a traditional two-category (cloud and rain), two-moment (mass and number) approach, we also compare AMP and BOSS with a single-category, four-moment setup, which avoids the artificial distinction between cloud and rain. Preliminary results show that the single liquid category greatly improves accuracy when predicting raindrop radius and rain rate.
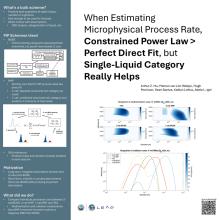
Hu-Arthur-poster.pdf
(727.41 KB)
Meeting homepage