Statistical techniques to constrain parametric uncertainty applied to the simulated radiative effects of biomass burning aerosol
Hamish
Gordon
Carnegie Mellon University
Poster
Our ability to simulate aerosol effects on clouds and radiation is critical to understanding aerosol effects on the climate system. Multiple sources of uncertainty limit the likely accuracy of calculations of aerosol radiative forcing. The representations of clouds and aerosols in climate models contain many uncertain parameters. Uncertainty in other quantities, such as emission rates of chemical and aerosol species, can also be represented by introducing parameters to scale the rates within their uncertainties. The `parametric uncertainties’ in these numbers are relatively tractable to study and constrain. It is possible that parametric uncertainties considered all together can represent a sizeable fraction of the overall uncertainty in aerosol-climate interactions.
To examine the relative importance of uncertain parameters and constrain them using observations, an established procedure is to perturb parameters simultaneously within estimates of their uncertainties over an ensemble of model simulations and then examine the sensitivity of variables such as radiative fluxes or forcing to the parameters.
A popular approach to constraining uncertain parameters in models using observations is `history matching’, wherein parameter combinations are excluded if they lead to values of an observable, for example aerosol optical depth, that are deemed to be inconsistent with observations. However, un-parameterized sources of error in simulations or observations may lead to substantial model-observation discrepancies which complicate the interpretation of constraints. I will present efforts to address this difficulty. We aim to maintain straightforward and statistically robust interpretations of any constraints on input parameters and on output variables that we can achieve. Following preliminary work documented by Carzon et al (Env. Data. Sci. 2023), we are applying our procedure to constrain the radiative effects of biomass burning aerosols simulated by the Met Office Unified Model over the southern Atlantic ocean using satellite and aircraft measurements.
To examine the relative importance of uncertain parameters and constrain them using observations, an established procedure is to perturb parameters simultaneously within estimates of their uncertainties over an ensemble of model simulations and then examine the sensitivity of variables such as radiative fluxes or forcing to the parameters.
A popular approach to constraining uncertain parameters in models using observations is `history matching’, wherein parameter combinations are excluded if they lead to values of an observable, for example aerosol optical depth, that are deemed to be inconsistent with observations. However, un-parameterized sources of error in simulations or observations may lead to substantial model-observation discrepancies which complicate the interpretation of constraints. I will present efforts to address this difficulty. We aim to maintain straightforward and statistically robust interpretations of any constraints on input parameters and on output variables that we can achieve. Following preliminary work documented by Carzon et al (Env. Data. Sci. 2023), we are applying our procedure to constrain the radiative effects of biomass burning aerosols simulated by the Met Office Unified Model over the southern Atlantic ocean using satellite and aircraft measurements.
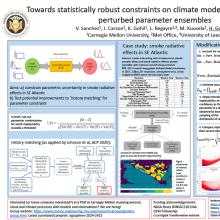
gordon-hamish-poster.pdf
(1.4 MB)
Meeting homepage