A Pattern-aware Feedback Framework for Climate Responses based on Green’s Function Experiments
Parvathi
Madathil Kooloth
Pacific Northwest National Laboratory
Poster
We devise a pattern-aware feedback framework for representing the forced climate response using a suite of Green’s function-based solar radiation perturbation experiments. By considering the energy balance at the top-of-atmosphere, a comprehensive linear response function (LRF) for important climate variables and feedback quantities such as moist static energy, sea surface temperature, albedo, cloud optical depth, lapse rate etc., is learned from the Green’s function data. The learned LRF delineates the efficiency of the energy diffusion in both the ocean and atmosphere, and the pattern-aware feedbacks from the aforementioned radiatively active processes. The LRF can then decomposed into forcing-response mode pairs which are in turn used to construct a reduced-order model (ROM) describing the dominant dynamics of climate responses. These mode pairs capture nonlocal effects and teleconnections in the climate and thus, make the ROM apt for capturing regional features of climate change response. A key observation is that the most excitable mode of the LRF captures the polar amplified response of the climate system due to doubling of CO2 and this mode is explainable in the data-learned pattern-aware feedback framework. The ROM can be used for predicting the response for a given forcing and for reconstructing the forcing from a given response; we demonstrate these capabilities for uniform forcing and forcing due to doubling of CO2.
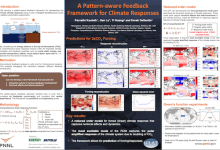
kooloth-parvathi-polar-poster.pdf
(7.04 MB)