Self-Adaptive Optimization of Ocean Observing Networks
John
Park
Old Dominion University
Poster
This presentation suggests the self-learning digital twin of ocean-observing networks by actively removing the uncertainties as multimodal heterogeneous sensors are available. Based on online machine learning for utility maximization, the new adaptive observation strategy will be computed on the fly. The knowledge discovery of observed environmental and unobserved (unmeasured) variables will improve the prediction accuracy. Graphical deep learning will integrate multimodal sensory sources of image and in-situ sampling by systematically reducing uncertainties. The poster will present a prototype for active sensing of hurricane eyes funded by NSF CISE Information and Intelligent Systems and NASA’s Jet Propulsion Laboratory and its application to ocean observation systems. The prior work will show how online updates from sUAS-collected meteorological data would benefit hurricane intensity forecasting, considering the temporal variation of uncertainty. Unobserved heterogeneity and randomness of the data have shown multiple modes of probability distribution in each location. A huge collection of multiple sources of granular microscopic data in each location may result in the loss of multivariate information if not retrieved properly. It is important to quantify the uncertainty of prior belief and update posterior when critical observations are obtained. However, traditional entropy theory cannot handle i) sequential learning multimodal multivariate information, ii) dynamic spatiotemporal correlation, and iii) the importance of observation for posterior approximation. In this paper, we advance autonomous in-situ sensing under highly uncertain and turbulent flow, analyzing the similarities between the different types of mixture distributions and allocating a cluster to each group across high dimensional time and space. Extensive experiments on hurricane ensemble forecasting data demonstrate the superior performance of our method over state-of-the-art baselines across various settings.
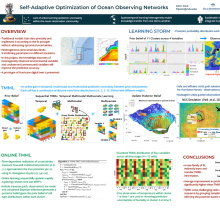
Poster file
Park-John-poster.pdf
(5.4 MB)