Parametric sensitivity of E3SM in the presence of aleatoric, observational, and structural uncertainty
Pappu
Paul
University of Illinois Urbana Champaign
Poster
The atmospheric component of the Energy Exascale Earth System Model (E3SM) contains numerous new characteristics in the physics parameterizations. It becomes quite difficult to comprehend the behaviors of the models and to tune the parameters due to the complicated nonlinear interactions among the additional elements. To better understand the model behaviors and physics, we plan to conduct 263 short simulations (3 years) in which 45 parameters carefully selected from parameterizations associated with cloud processes, convection-precipitation, and aerosols were perturbed simultaneously using the Latin hypercube sampling method. As Climate models are too expensive to undergo iterative optimization procedures, a machine learning emulator is used that can map model parameters to the subset of model output. In this study we use our 263 ensemble members to train and test the Gaussian Processes, Random forests, Convolutional Neural Net (CNN) emulator. This computationally efficient emulator is then used to obtain an estimate of the optimal value of the parameters that best matches observations when comparing a subset of model output.
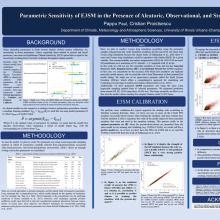
Paul-Pappu-poster.pdf
(713.59 KB)
Meeting homepage