Updating observational benchmarks for rainfall to test model forecasts
Anna Lea
Albright
Harvard University Center for the Environment
Poster
Given advances in machine learning-informed models for weather prediction, higher resolution storm-resolving models, and interest in improving climate predictions, a question that comes to the fore is how to examine skill? For purposes of evaluating climate predictions, it is necessary to develop long, homogenized datasets that accurately represent trends, which can be difficult to obtain on account of changes in instrumentation and measurement protocols. In this work we seek to expand upon existing observational benchmarking efforts for weather and climate, with a focus on rain. Our approach is to develop a large set of observed covariates for rainfall features and examine which are the most robust to inhomogeneities and informative with respect to testing skill in climate predictions. Extending the temporal interval further back in time, as well as to present-day is useful for generating a long enough baseline for testing climate forecasts. Candidate statistics include the width and location of, and rainfall intensity within the Intertropical Convergence Zone over land and ocean; diurnal and seasonal variability in rainfall accumulation for different climate zones; and dichotomized statistics of rainfall estimated from both rain gauges and satellites. An ensuing question is how variability in these statistics can be explained by climate forcings, internal variability, and observational error.
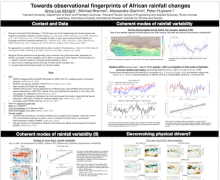
Poster file
albright-annalea-confronting-poster.pdf
(3.73 MB)