Detection of anthropogenic influence on regional precipitation trends using large ensembles and machine learning
Gavin D.
Madakumbura
University of California - Los Angeles
Poster
Precipitation constitutes a fundamental component of the Earth's climate system. The identification of forced components in observed precipitation records is critical for evaluating the contribution of anthropogenic climate change on global and regional hydrology. While existing signal detection methods have effectively identified a discernible human influence in the observational records of precipitation, they have predominantly focused on a single time-invariant pattern, such as the global mean or the zonal mean profile. Thus, the temporal evolution of the forced precipitation response in individual regions is not well quantified. This study addresses this gap by applying a 3D Convolutional Neural Network (CNN) to annual precipitation data from five large ensembles of climate model simulations. The CNN incorporates spatial dimensions (latitude-longitude) as well as a temporal lag to model the forced response of each grid cell. The algorithm uses a synthetic dataset generated by overlaying the forced trend and internal variability component of each large ensemble, resulting in 25 distinct large ensemble datasets. This novel approach demonstrates robust performance during out-of-sample testing on models not included in the training process. Subsequently, various observational datasets are introduced to the trained CNN to extract the historical observed forced component. The results reveal a discernible forced signal along the tropical rain belt over the Pacific Ocean, Africa, and the Indian Ocean. Additionally, pronounced drying patterns emerge over the Amazon and Southern regions of Africa, features not easily identifiable in the simple trend estimates of the raw dataset. Finally, we use AMIP simulations to assess model performance and historical single forcing CMIP simulations (greenhouse gas, aerosols, natural forcing) to better understand the contributions of individual forcings to the regional patterns of forced precipitation change.
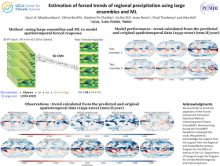
Poster file
madakumbura-gavin-confronting-poster.pdf
(1.12 MB)