Developing Observational Large Ensembles for climate variability
Historical records of temperature and precipitation contain clear evidence of a forced climate change signal. Superimposed on that signal is variability that is internally generated by the interacting components of the climate system. Interpretation of our historical records, as well as predictions for the future, requires modeling both the climate change signal and the internal variability. One way to do so is through the development and analysis of initial-condition climate model ensembles, which are designed such that the average across the ensemble provides an estimate of the forced signal, and the spread across the ensemble provides an estimate of the internal variability. While these ensembles are powerful tools, they suffer from known and unknown biases that can hinder their face-value interpretation.
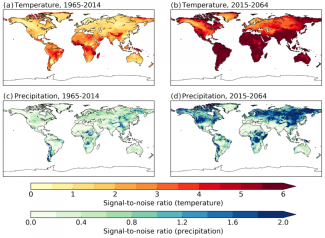
In two recent papers published in the Journal of Climate, McKinnon and coauthors propose a complementary approach to create ensembles for seasonal-average temperature and precipitation over land that can also be used to study internal variability. Rather than using a dynamical model, the authors developed a statistical approach that is informed by the historical observations. For this approach, temperature and precipitation are modeled as the sum of a best-estimate of the forced component, the response to three large-scale modes of sea surface temperature (SST) variability, and the residual “climate noise.” The ensemble is created by generating alternative versions of the time series of the three SST modes and the residual climate noise that are consistent with the spatiotemporal properties of the observations. The statistical methodology is tested in the context of the NCAR CESM1 Large Ensemble (CESM1-LE) and found to generally outperform CESM1-LE in simulating temperature and precipitation variability over land, particularly in the mid-latitudes. The statistical ensemble combined with an estimate of the forced component of temperature and precipitation change is termed the Observational Large Ensemble (Obs-LE).
The world we live in is one shaped by both anthropogenic influence and internal climate variability. In order to build communities that are resilient to weather and climate variability, it is necessary to accurately model both the human and natural contributions, and to understand the envelope of uncertainty around our projections. These goals can best be accomplished by optimally merging insights from dynamical models and the observational record.
Internal variability and regional climate trends in an Observational Large Ensemble (Journal of Climate)
An 'Observational Large Ensemble' to compare observed and modeled temperature trend uncertainty due to internal variability (Journal of Climate)
1University of California, Los Angeles
2NCAR
3Carleton College
4University of Bergen